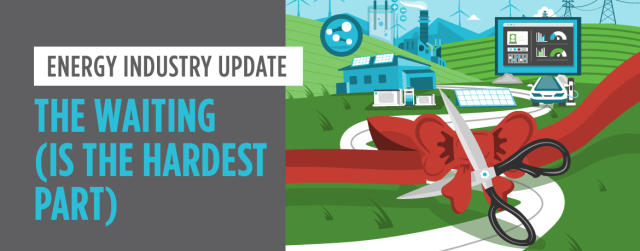
On the journey to value creation in the HR analytics space, organizations have to move past the historically focused reporting activities and begin to conduct more creative and predictive analyses.
One company that has fully matured in the predictive analytics space is Amazon. Amazon’s success in customizing views, based on shopping history and site clicks, gives the impression it knows more about our consumer behaviors then even we know ourselves. Amazon has harnessed the power of predictive analytics to the extent that it is now patenting “anticipatory” shipping —a method to start the delivery of packages even before customers click “buy.” Amazon is moving beyond just predicting what we want. Soon, that special product will be in route to our homes and businesses before we even know we want it.
HR has the potential to gain similar insights with talent data, such as predicting when talent has the potential to depart from the company or determining when leaders need to achieve particular competencies to be successful long term. Recently, ScottMadden performed a first-year turnover analysis for a client to help the new HR analytics organization achieve an early win. We were asked to build a model to predict which employee constituents would have the highest first-year turnover and which internal or external factors would have the greatest impact on turnover. Using this model, we conducted scenario-based testing (i.e., if we pulled one lever, such as decreasing span of control, we expected a certain impact to turnover).
So, where do you start when asked to do this type of analysis? As we’ve stated in, “Predictive Analytics: Building the Infrastructure,” analytics organizations have to understand the business need. What is driving this request? What are they seeing? In addition, companies need to understand the data (i.e., the test population) through descriptive statistics: Are there explainable trends in first-year turnover (e.g., seasonality)? Are there certain jobs turning over more quickly than others? Discussing these elements with the business can give some insight to help craft your initial hypotheses.
Next, analytics groups should prepare the data to do the analysis. For our client, we modeled turnover against internal variables, such as manager span of control, manager and employee performance, and orientation hours. We also included external variables such as employment rate, number of graduates in a certain field, and industry performance indices.
It should be mentioned that this kind of analysis is substantial, requiring significant data refinement and model iteration. Even after you prepare the data based on what you learned from the business and understood from the original situation, you could possibly uncover learnings that will require you to add additional data elements and restart the analysis. One framework that HR analytics organizations use to demonstrate this iterative process is the Cross Industry Standard Process (CRISP ) data-mining codification. Figure 1 shows the iterative steps between data preparation and the modeling steps mentioned above.
To be successful, coming to the analysis free of bias is important. When embarking on organizational analyses, we, as humans, cannot help but bring our own preconceived, biased notions of what we think the outcome will be. We are rooted in belief systems about how things work and how we think people will respond. This is a cultural change for many—especially those who may have worked in HR for a long time and may have deeply rooted beliefs about why turnover, for example, may be occurring. What is both exhilarating and challenging about Big Data-driven analysis is that you may arrive at a finding that completely contradicts what was expected, or conversely, you may find the data do not substantiate the original hypotheses, so correlations between data points thought to be meaningful are statistically insignificant.
In addition, you should not over-engineer the model. With the massive amount of information available from data systems and after multiple iterations of analyses, you could easily fall victim to an overabundance of information. However, at this point, it is important to remember that not everything that counts can be counted and not everything that can be counted counts. Keep true to your original goals of the model, keep it as simple as possible, and let the data prove or disprove the hypotheses. You cannot force results—true HR analytics is an ongoing exploration and not a guaranteed destination.
At ScottMadden, we work with many companies to set up or improve their analytics functions and close the gap between aspiration and ability. For some, this first analysis can be difficult, but we have seen it be successful if you start with quick wins and are diligent and persistent in your approach.
View MoreSussex Economic Advisors is now part of ScottMadden. We invite you to learn more about our expanded firm. Please use the Contact Us form to request additional information.